Hello, my name is Peter Biggle. I'm chairing today's RSS webinars. I believe well, I've got a throne on the line! Hello, Brad! Hi, Peter! And we have Andrew Gelman lined up as our invited discussant. But I would like to encourage anybody from the audience who wants to join in the Q&A session at the end. You can either write your question, in which case I'll read it out for you at the end of the presentation. You need to use the Q&A icon at the top of the screen. Or, if you do want to actually speak directly, then you can press star on your phone to un-mute yourself. Please do remember to mute yourself when you've finished. I should say, we're grateful to Quintiles for their generous sponsorship of this series of journal webinars. And I'm particularly grateful to HQ staff Jack and Judith for all the work they've been doing behind the scenes. It's a great pleasure to introduce Brad Efron as today's speaker. He's extremely well known all around the world and is one of our most distinguished statisticians. But, in particular, I think everyone would recognize that Brad, in particular, was doing data science a long time before the term was invented. And he's always been one of the real deep thinkers about the interface between statistics and computer science and the implications of that to the future of our discipline. And I'm sure he won't mind me mentioning that he has a book in preparation with Trevor Hasty with the captivating title "Computer Age Statistical Inference". And I, for one, am looking forward to that very much. But without further ado, Brad, if you're ready to go, you'll be in charge of the presentation, and then we'll move to Q&A when you finish....
Award-winning PDF software
Video instructions and help with filling out and completing What Form 5495 Estimated
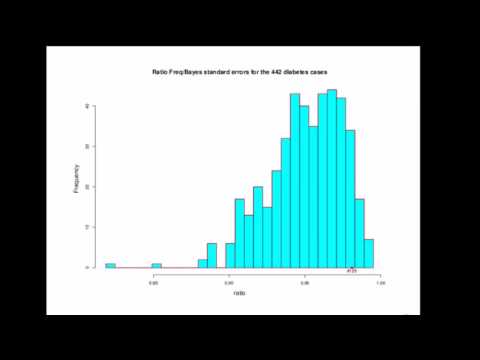