Hello, so to continue with our pattern recognition, the first two lectures we have gone through a general overview of pattern classification. We looked at what is the problem of pattern classification. We defined what pattern classifiers are. Now we have looked at the two block diagram model. That is, given a pattern, you first measure some features, so the pattern gets converted to a feature vector. Then the classifier essentially maps feature vectors to class labels. So, as I said, the course is about classifier design. We looked at a number of classifier design options as an overview. Now, from this lecture, we'll go into details. So, just to recap what we have done so far, we've gone through a general overview of pattern classification. A few points from the general overview that I would like to emphasize here is that a classifier that we have already looked at is the Bayes classifier. The base for the Bayes classifier is taking a statistical view of pattern recognition. Essentially, what it means is that a feature vector is essentially random. So, the variations in feature values when you measure patterns from the same class are captured through probability densities. And given all the underlying class conditional densities, we see that Bayes classifier minimizes risk. We saw the proof for the only time is minimizing problem in classification. We will see the general proof of this class. So, Bayes classifier essentially puts a pattern in the class for which the posterior probability is maximum, and it minimizes risk. If you have the complete knowledge of the underlying probability distributions, then Bayes classifier is optimal for minimizing risk. There are other classifiers. For example, we have seen nearest neighbor classifier among the other classifier. We will come back to nearest neighbor classifier...
Award-winning PDF software
Video instructions and help with filling out and completing Form 5495 Minimizing
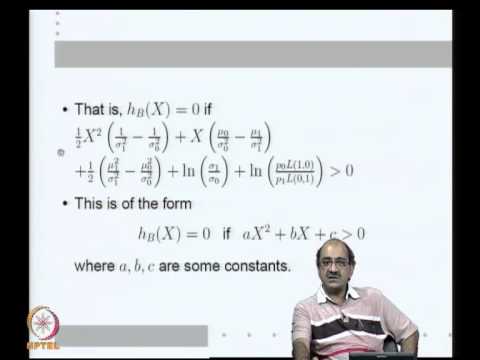